A common fear about Artificial Intelligence (AI) is that it will one day make human workers obsolete. If we had to name one area where this idea is patently wrong and grossly misplaced, it would have to be healthcare.
Staffing shortages in the healthcare industry are a global phenomenon. All countries, regardless of their level of socio-economic development, suffer from it to some degree. WHO estimates indicate that there will be a shortfall of 18 million trained professionals in the global healthcare sector by 2030.
The burnout caused by two years of the COVID 19 pandemic is making things even worse. Physicians, nurses, and diagnostic technicians need all the help they can get to provide timely care to those in need.
Medical AI has a massive role to play here, with the potential to reduce the burden on hospital staff, speed up critical processes like diagnosis and data management, and develop new treatments/drugs. But there is a massive bottleneck – medical data labeling.
What is AI Data Labeling – A Quick Primer
We create and train AI with the ultimate purpose of reducing human workload in labor-intensive, monotonous, or potentially dangerous tasks. Yet one of the great ironies of AI development is that the process in itself is incredibly labor-intensive!
Machine learning (ML) is an important part of AI data science. It focuses on providing AI software the ability to automatically learn and improve certain skills without constant human intervention. To make this happen, AI developers need vast quantities of data.
Depending on the skill you want to teach the AI, this data could be anything – images, videos, audio files, or text. Crucially, you cannot simply feed raw data to the AI algorithms. They need to be properly annotated using best-in-class annotation tools, and labeled with meaningful and informative tags to provide some reference or context to the machine AI.
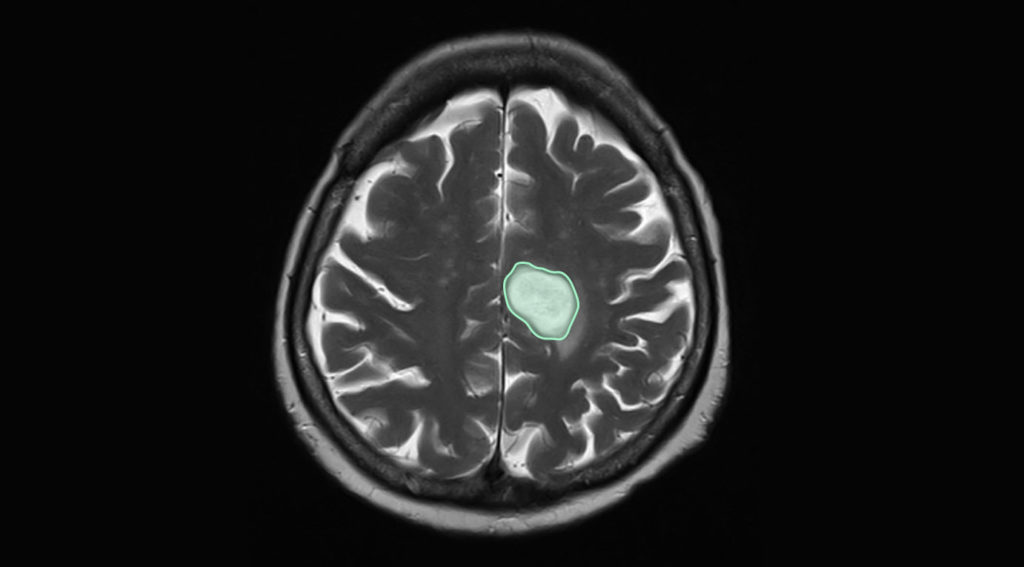
Take an example of a machine learning algorithm project to teach AI to detect malignant tumors from patient X-rays. The raw images do not hold any meaningful information for an untrained AI. Data labelers provide the context in the following ways:
- Grouping images into broad categories – ones with and without tumors, for example
- Provide further segmentation – classify different stages or types of tumors
- Provide granular data – mark out specific pixels to identify tumors
For maximum efficiency in machine learning, the AI needs to look at as many annotated data sets as possible. You have to cover a wide range of real-world scenarios with these data sets to improve AI accuracy and ML model performance when identifying a tumor from a raw image in a clinical setting.
This is easier said than done in the chaotic world of medical data.
Why Healthcare Data is a Minefield for AI Research
AI research has many exciting branches – Natural Language processing to look at texts, Computer Vision to decode images and video, and Audio Processing to process sounds, speech, and more. They all have varying levels of applicability and automation application in medical AI research.
But as we have already noted, you need vast quantities of high-quality annotated data to get the best out of any medical AI project. While quantity is not a major issue as far as healthcare data is concerned, quality is another story altogether. IBM learned this the hard way.
Barely a decade ago in 2011, the tech giant made an ambitious push into healthcare with its famous Watson supercomputer. The AI had made headlines beating humans in the popular TV game show Jeopardy. IBM had big plans for Watson in the medical industry, focusing on cancer treatment.
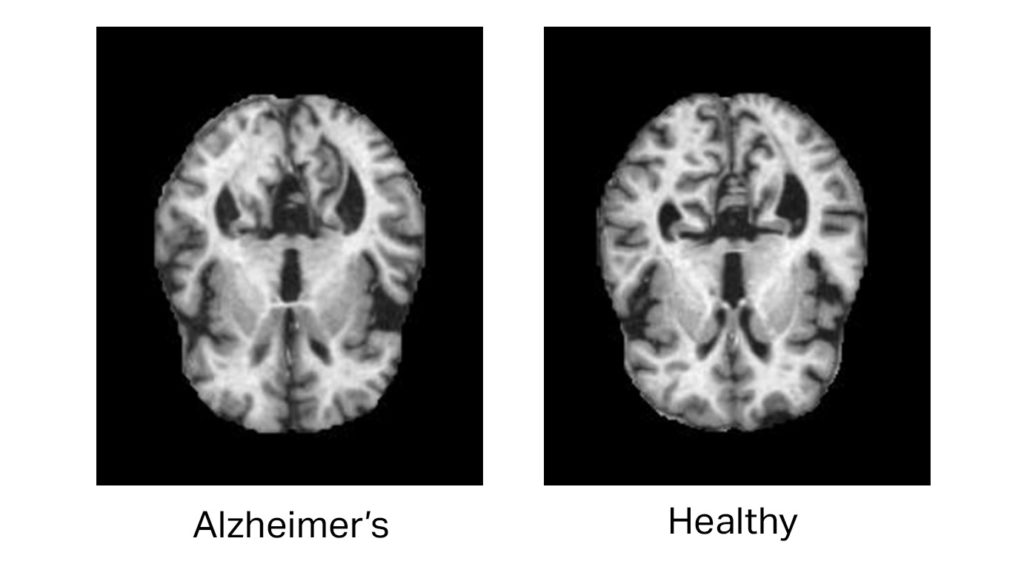
Despite access to vast troves of data from numerous high-profile partners, the Watson project failed to make any headway. Several reasons have been cited, and they all revolve around the nature of healthcare data. Modern health systems generate vast troves of data that suffer from the following issues:
- Heavy fragmentation due to the use of multiple software platforms and data silos
- Lack of digitization, with lots of data still languishing in paper form
- Inherent complexity in language and medical terminology
Despite having formidable natural language processing skills, the Watson AI failed to make significant headway due to the chaotic nature of medical data it had to deal with. The cost of converting raw text data into meaningfully annotated and segmented data, was prohibitively high, bordering on the impossible.
Nonetheless, the failure of Watson AI served an important purpose – it showed us the limitations imposed by healthcare data on medical AI development. Consequently, the focus has shifted away from a broad, ambitious approach to more focused, practical solutions.
For instance, NLP holds great promise in training chatbots and virtual assistants for patients. Data extraction and semantic annotation can empower the AI to interact with patients suffering from degenerative neurological disorders, assisting in the assessment and monitoring of conditions like Alzheimer’s.
Outside NLP, a focused approach to another discipline in machine learning holds a lot of promise for medical AI – Computer Vision.
The Explosive Potential of Computer Vision in Medical AI Data Labeling
Unlike patient records and other textual data, medical images are more platform agnostic and universal. There is relatively less cleanup required when dealing with X-ray, CT Scan, MRI, and Ultra Sound images/videos.
And they are also quite plentiful. According to EMC and IDC research, hospitals across the globe generate more than 50 petabytes of data annually, with 90% made up of medical images. And unlike patient health records, sanitizing/anonymizing these images is a much simpler task.
This mountain of image data also represents a major pain point for many health systems – fast and efficient diagnosis. There is an acute shortage in the number of qualified radiologists in major healthcare systems in developed countries like the USA, Australia, and the UK.
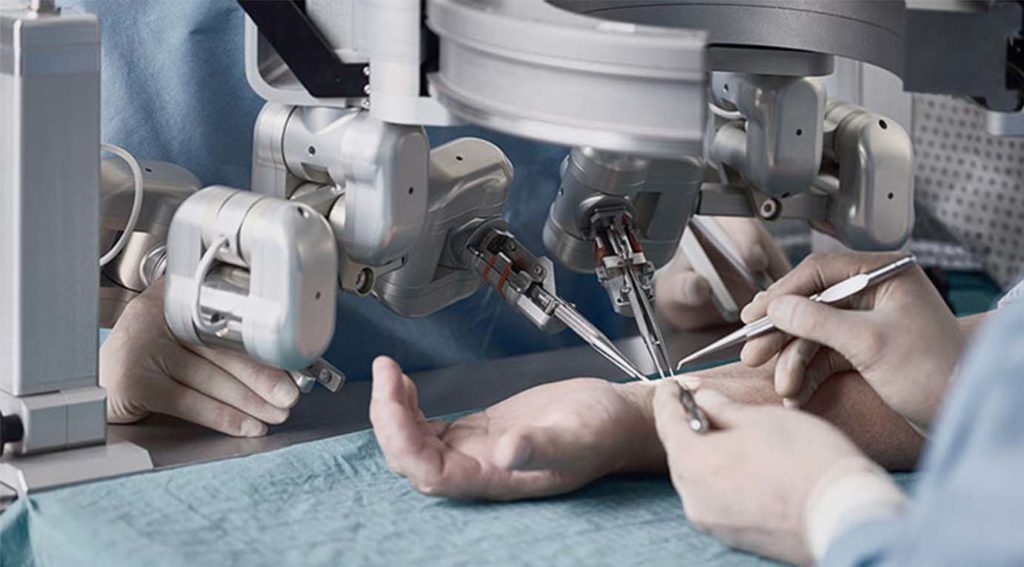
The Association of American Medical Colleges predicts a shortage of nearly 122,000 radiologists by 2032. In the National Health System in the UK, 98% of radiology departments fail to complete diagnostic commitments in contracted hours due to staffing issues, according to the Royal College of Radiologists.
Across the globe, critical care is being delayed as patients await imaging reports. The situation is being exacerbated by the increasing demands of an aging population, as well as the recent impact of the coronavirus.
AI trained in medical image diagnostics has the potential to make a difference here, providing vital assistance to overworked radiology departments. With the ability to work round the clock without tiring, they can take some of the workloads away from the human experts.
Precision robotic surgery is another exciting field where training video datasets with pixel-level annotation can make massive strides possible. Using tags and labels on critical organs, lesions, and other pathologies across millions of frames, researchers can create robotic AI that is safe, accurate, and a reliable assistant for surgeons.
However, behind all this thirst for annotated data lies a major catch, or rather, a catch-22 situation.
The Catch-22 involving Medical Data Labeling Experts
There are numerous use cases of diagnostics where AI has shown significant promise. With rich annotated training data, AI can be taught to detect early signs of lesions that predicate neurological disorders like Alzheimer’s faster than the human eye.
Polygon annotation has utility in helping AI analyze complex structures like teeth, which are very useful in dentistry. Annotated X-ray images and bone CT scans can help find hairline fractures in the elderly that are not easily visible to human operators.
Bounding box annotation is essential for the accurate detection of kidney stones, while semantic segmentation annotation helps train the AI to detect prostate cancers from imaging data. All these image labeling tools require one common factor – a labeler with domain expertise.
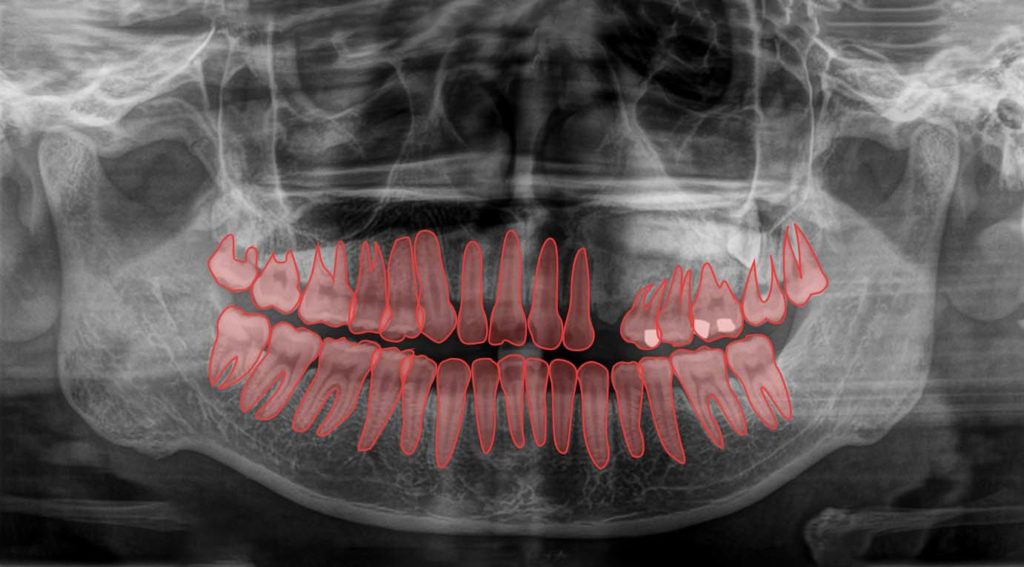
Radiologists undergo rigorous training for 3-4 years to acquire the domain expertise necessary to make judgment calls on scan results. They are essential to ensure that annotated data sets are of high quality and objective accuracy, especially when dealing with edge cases that are susceptible to misdiagnosis.
In a perfect world, AI firms would employ professionally certified radiologists and experienced radiographers to handle all medical image data annotation projects. As already noted, this is neither feasible nor affordable in a modern healthcare market where the experts are in short supply at the most critical positions – on the frontlines of hospitals.
So, we have a Catch-22 situation – you need more accurate and reliable AI to assist the radiologists, but for that, you need more radiologists. However, as more AI developers look towards data labeling service providers for answers, new solutions to this problem have also come up.
Data Labeling Experts Can Take the Role of Medical Experts
Given the highly specialized nature of data labeling and annotation, most enterprises find it too resource-intensive for efficient scaling. As projects expand in scope, the cost of creating in-house data sets can increase exponentially.
It requires the right mix of technologies, processes, and people to deliver high-quality machine learning data. In a typical AI project, the data annotation and labeling can take up 80% of the development time if left in-house.
This is why enterprises like iMerit exist. We focus on the heavy lifting involved in data segmentation, sanitization, and annotation. AI developers are free to focus on their core competencies, no longer worried about the shortage of qualified labelers.
One of the key challenges to data labeling is workforce training and management. As a specialized enterprise, iMerit can focus all its resources on creating the “right kind” of medical data labeling experts.
In a pathbreaking study involving our annotation SAAS partner Labelbox, the researchers from London’s Imperial College established that trained labeling teams can match the quality of annotations created by certified medical professionals.
The task involved annotating fetal ultrasound imagery for signs of congenital hypoplastic left heart syndrome. The two data sets in the study created by labeling professionals and medical professionals were similar in quality.These results challenge a common assumption that only trained radiologists are qualified enough to create annotated medical image data sets for AI machine learning models. But this does not mean that data labeling services can take a cavalier approach to their workforce involved in medical AI projects.
The Importance of Selection Standards, Training, and Oversight
In most developed economies, the healthcare sector attracts the strictest regulations related to patient medical records privacy, data protection, and overall product safety standards. Low-quality data sets will adversely affect the accuracy and reliability of AI and increase risks of non-compliance.
To avoid this data labeling service providers must focus on the following aspects while working on medical data:
Domain Expert Oversight:
There is no way to avoid this aspect – a qualified medical expert is essential for the successful creation of reliable AI medical datasets. The domain experts at iMerit function in the role of a Solution Architect – working closely with clients to create guidelines for labeling teams.
They also provide quality oversight during the labeling workflow, handling queries from labelers related to edge cases and other areas of confusion that may arise from raw image data. The Doman Expert is the vital bridge between clients and our image annotation teams.
Candidate selection:
Here at iMerit, our team of data labeling experts has 100 members, carefully chosen based on selection criteria involving educational qualifications, aptitude for video/image annotations, pattern recognition, and agile learning capabilities. Only candidates that exceed a high skill threshold are selected for medical labeling teams.
Project Specific Training:
For best results, medical labeling specialists undergo two rounds of training. The first is a domain-specific round that brings them up to speed on essential aspects like medical terms, pathologies, patterns, data manipulation, etc. This intense curriculum provides a grounding in medical conceptual knowledge.
The second round of training is client/project-specific. The in-house medical expert provides additional training based on inputs from the client to ensure that the image labeling team has all the tools and project parameters to deliver labeled data sets that match the client’s AI project requirements.
Conclusion
The global healthcare systems face an uncertain future. The combination of future pandemics, the ever-increasing demands posed by a demographic shift to an aging population, and increasing labor shortages all combine to create an additional burden for healthcare professionals.
Medical AI can provide the advantage of speed, lower costs, and increased efficiency. But only if they are trained using high-quality annotated data sets. Only data labeling service providers that rely on a combination of domain experts, trained specialists, and other best practices can deliver this.
iMerit is an industry leader in labeling, annotation, segmentation, and transcription, and analysis of diverse data sets – images, text, video, audio, LIDAR, and more. We have extensive experience in providing specializing segmentation and annotation services across 20 million data points for the healthcare sector.
Contact our experts today to learn how iMerit can create rich data sets for your AI project.