AI and Machine learning is being applied to multiple aspects of insurance practice since the industry is full of bottlenecks and manual processes such as claims processing, underwriting, policy administration and customer service. According to a McKinsey study, 25% of the insurance industry will be automated in 2025 thanks to AI and machine learning techniques. Here are various facets of the Insurance industry which are benefitting from AI and Machine Learning.
Risk Assessment
Detecting risks early in the process enables insurers to make better use of underwriters’ time and gives them a huge competitive advantage. Machine learning and AI can provide insights right at the time of quote by detecting property features such as roof condition, type, and shape as well as common risk factors like fire hazards, overhanging trees and existing damages. Insurers can also easily prevent fraudulent claims of damaged property during disasters.
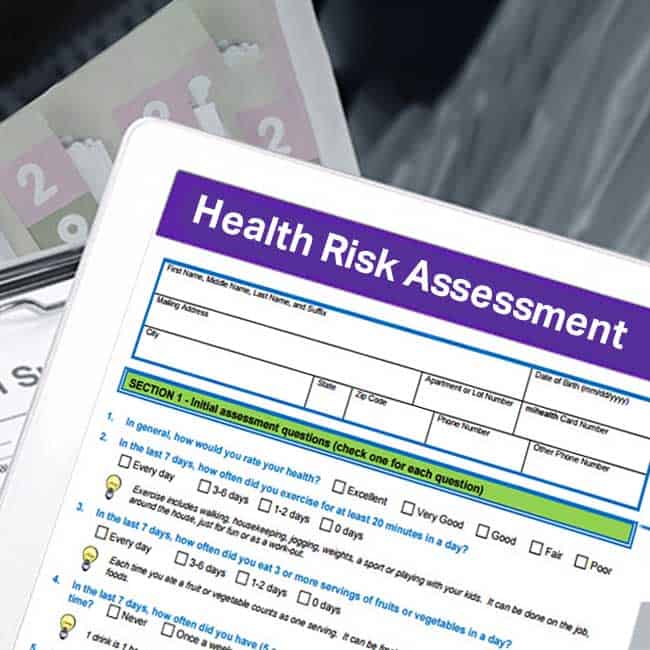
According to the FBI, non-health insurance fraud in the US is estimated at over $40 billion per year, which can cost families between $400–700 per year in extra premiums. Machine learning can be used to improve insurance companies’ risks and actuarial models, which can potentially lead to more profitable products.
Example – Corvus Insurance implements AI technologies in its risk prediction platform to help insurance brokers better anticipate losses and better protect policyholders.
Data Annotation: Data Extraction and Data Verification
Damage Detection and Assessment
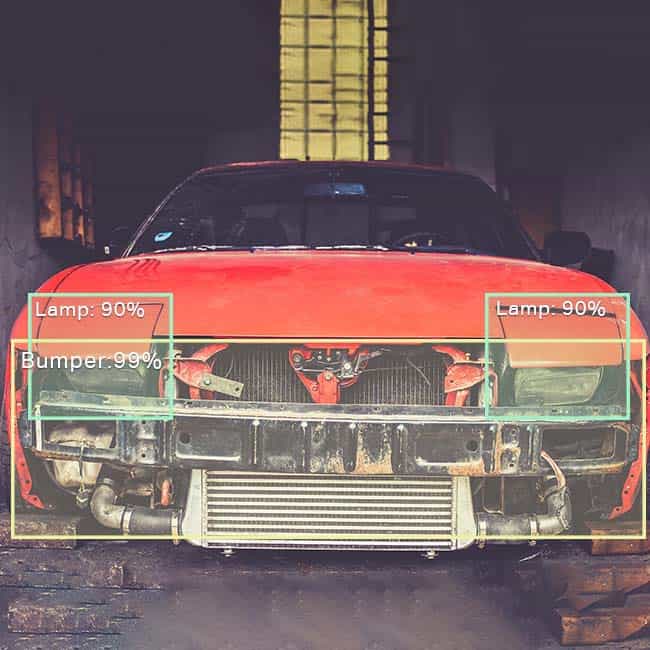
The type, location and extent of vehicle and property damages can be assessed by machines to estimate the repair cost faster and set the right insurance claims amount accordingly. Paired with aerial imagery, machine learning technologies can identify hail strikes on roofs and detect random patterns to help carriers avoid paying fraudulent claims.
Example – During the California wildfires of 2017, EagleView helped insurance carriers determine the total number of structures damaged or destroyed.
Data Annotation: Image and Video Annotation to detect and analyze damages
Automated Underwriting Verification
Automated insurance underwriting utilizes advanced AI and ML technology in tandem with the insurance company’s underwriting guidelines to determine whether or not to accept the risk presented by the client. This, in turn, allows providers to generate a profit from underwriting and enhance customer satisfaction through more personalized policies. Data from customer’s credit history, insurance telematics, and other personal data like driving records, insurance applications or even social media can be extracted to generate automated claim decisions and applicable interest rates. Fitness and vehicle tracking systems in both the health and auto insurance sector give rise to the dynamic, intelligent underwriting algorithms that cleverly control the way premium is dictated.
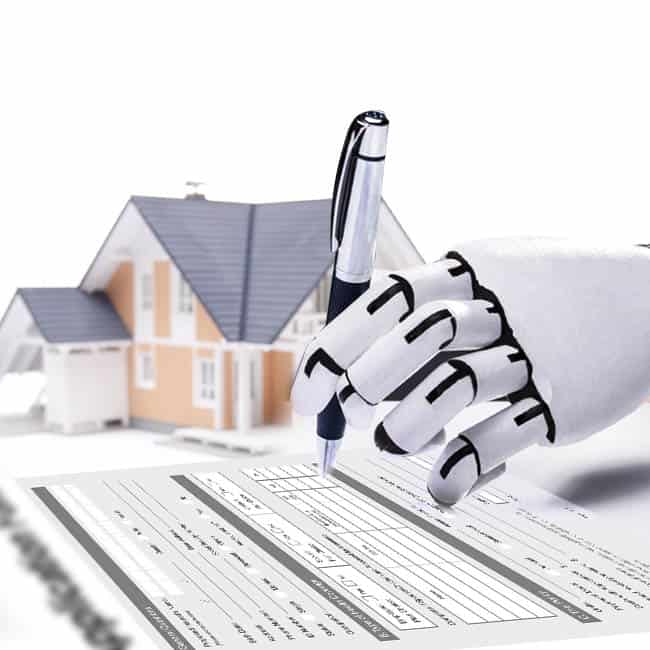
Example – MetroMile, a US-based start-up, has established such a dynamic underwriting system known as ‘pay-per-mile’ where usage of a car determines the insurance premium. Here, an AI-based device installed on the vehicle by the company uses a special algorithm to monitor miles, jerks, collisions and frictions, speed patterns and other car struggles on the road, and it collects detailed data essential to decide whether or not drivers deserve low premiums.
Data Annotation: Data Extraction and Data Verification
Claims Processing
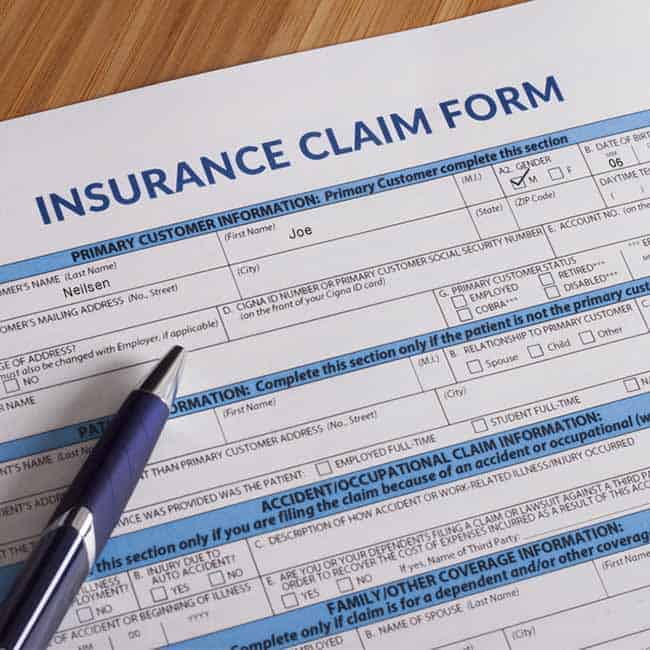
Typically, claim processing takes several days as insurance agents have to gather and check data from multiple sources. Insurers are now using machine learning to improve operational efficiency, from claims registration to claims settlement. The automated insurance claim process can remove excessive human intervention and can report the claim, capture damage, update the system and communicate with the customer all by itself.
Example – Kirontech claims that its software KironMed uses machine learning to analyze medical claims and detect patterns that may signify health insurance fraud or waste (underutilized services).
Data Annotation: Named Entity Recognition and Entity Classification
Customer Support
To improve customer experience, many insurers are investing in virtual assistants like chatbots. The chatbot frontier is bound to expand, and companies that leverage AI-driven customer data for chatbot support will flourish far into the future. The best insurance chatbots would be able to hold a conversation using natural language and help the customer complete the process while providing personalized suggestions to lower the quote and guiding the customer from start-to-finish.
According to a Juniper Study, the use of conversational AI chatbots for insurance will lead to cost savings of almost $1.3 billion by 2023, across motor, life, property and health insurance, up from $300 million in 2019.This can prove to be a differential factor that can allow insurance firms to stay at the forefront of the competition.
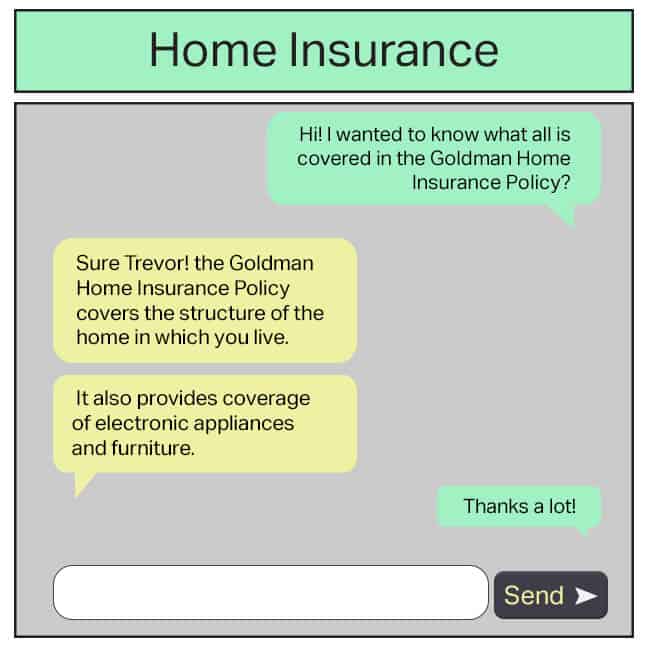
Data Annotation: Natural Language Processing Services
Example – Lemonade, a peer-to-peer online property and casualty insurer, heavily relies on its app based chatbots. Backed by AI, the chatbots can craft personalized insurance policies and quotes for customers right in the Lemonade app, and respond quickly to a variety of customer queries and process claims.