At the iMerit ML Data Ops summit, iMerit’s Director of Medical AI Sina Bari interviewed Bobby Anderson, Clinical Insights Leader at GE Healthcare, Daniel Chow, Co-Director at the UCI Center for AI in Diagnostic Medicine, and Naqi Khan, Principal Program Manager for Health AI at Microsoft.
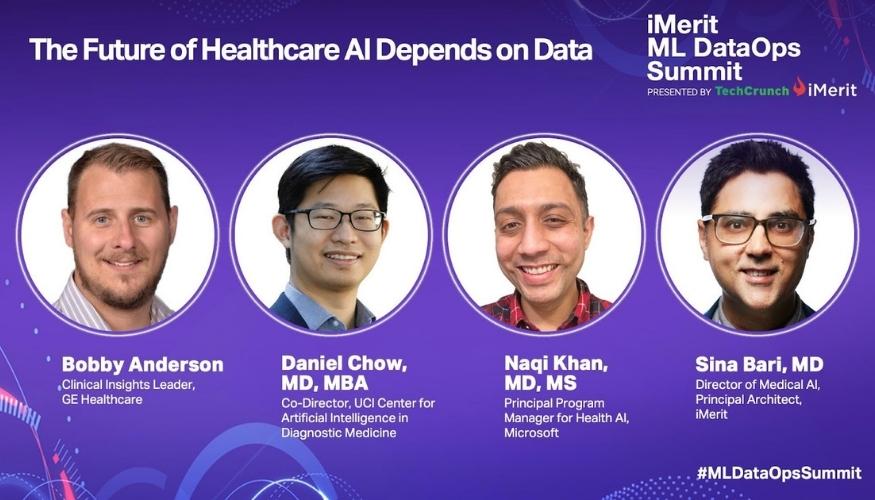
This panel of experts talked about the latest developments, challenges and adoption strategies of AI in the medical space. Specifically, the group discussed:
- The importance of high-quality labeled data
- How clinicians function as humans-in-the-loop
- How annotation tooling impacts clinicians and practitioners
AI Adoption is the Future
While AI is certainly the future for medical technology, the healthcare industry simply will not tolerate technologies with higher error margins than a human. Especially by having a lot of use cases in diagnostics, AI still needs to increase its performance before widespread clinical adoption can become a reality. Current proof-of-concepts have very promising results, such that every CTO is aware of the developments in the space and have the implementation of AI-based technologies on their roadmap.
Naturally, AI solutions require a lot of computing power that still isn’t widely available in smaller clinical practices. In the case of AI for ultrasound, the algorithm needs to process large amounts of data and perform measurements in real time. With more powerful devices available for clinicians or edge-computing solutions, AI diagnostics are currently restrained in a clinical practice.
What is Holding Back AI in Healthcare?
As with most AI projects, the largest challenge is the lack of high-quality labeled data. Considering the worldwide shortage of doctors and other trained staff, there is an inherent shortage of necessary medical expertise to create high-quality medical datasets.
With existing medical staff already stretched thin, the number of industry-trained data annotators is currently too low, making any advancements slow. In addition, data annotators must have use-case specific knowledge that applies to the data they’re annotating. Naturally, brain hemorrhage data is considerably different to lung cancer data. This problem is further exacerbated by the immaturity of tooling, which poses higher error rates and longer labeling times.
AI in healthcare also poses a number of edge cases. One major aspect that must be considered is that data used to train AI models must also reflect the population the model will be used on. For example, an AI model trained on data from regions such as south-east Asia or Africa may not be applicable to the US. Whereas in the former regions, the population may have malnutrition as a risk factor, the US population has an obesity risk factor. Another case is the definition of asymptomatic patients. An ER doctor has a different definition of asymptomatic compared to an IV Specialist, requiring AI developers to take into consideration all definitions and have experts to accurately label data.
“We took a COVID calculator from overseas and tried to apply it to the US population. It quickly broke down due to different population risk factors.”
– Daniel Chow, MD.
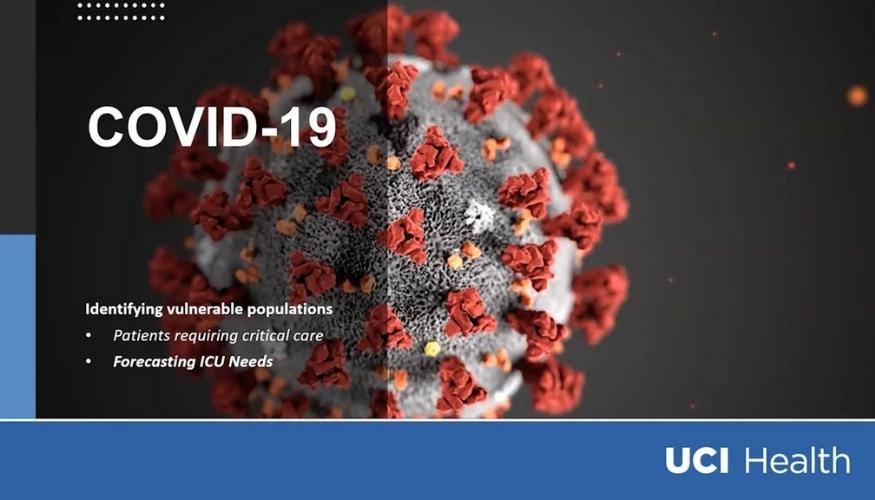
Improving AI Adoption
The biggest hurdle to AI adoption is the lack of trust in the technology. Therefore, AI developers must continually validate their applications as they apply them. In addition to validation, the tools must be built with the end user in mind. A tool used by clinicians will require that clinicians’ input – effectively making them the human-in-the-loop. They need to involve clinicians during the ideation phase. Simply asking clinicians about their problems and how they envision a solution has the potential to streamline development. They have the right insights and understand which metrics to look for and not.
A similar process should happen on the other side of the table, which entails getting data scientists and engineers to see their applications working in a real-world scenario. Arranging visits to see how the tools are used can help them see the impact of their work.
“We’ve put together teams with data scientists, physicians and ultrasound technicians, so that we have input at every step of the way from who will be the end-user.”
– Bobby Anderson
Future Developments
Our panel of experts believes that key developments in the area will include the ability to orchestrate datasets, an emergence of multiple AI vendors to enrich the ecosystem, and the augmentation of clinicians.
For dataset orchestration, we already have multiple complex data streams such as data from Omic disciplines, to electronic health records and to socioeconomic determinants. Mapping all these types of data together using traditional methods is borderline impossible. AI can fulfill a great use case here that will allow clinicians and researchers to integrate multiple types of datasets for better and more accurate predictions.
As the industry matures, we also expect to see more AI tools and companies entering the space. With a wider ecosystem, we will most likely see high degrees of interoperability and super-specialized AI tooling.
“We’re releasing tools that help clinicians perform their work better and more accurately, and not to take on so much of the administrative burden that traditionally caused clinicians to burn out”
– Naqi Khan
Augmentation refers to the enhancements of clinicians’’ abilities as opposed to replacing them. It is highly likely that clinicians will be just as important in their roles after mass AI adoption. Positioning developments in the area in this light will impact the medical staff’s reception of new tooling as a solution to current inefficiencies rather than as a threat to job stability.
Have you explored our cutting-edge Radiology Annotation Suite? Click here to discover its powerful capabilities.