At the iMerit ML Data Ops Summit, NLP and AI experts from Target, UC Davis, OpenCity, and The Floor met to discuss how new functionalities in natural language processing (NLP) are advancing the capabilities of artificial intelligence. These experts discuss the trade-offs between automated and human workflows, and suggest ways to enhance the data labeling process to improve data labeling efficiency and the quality of the data produced.
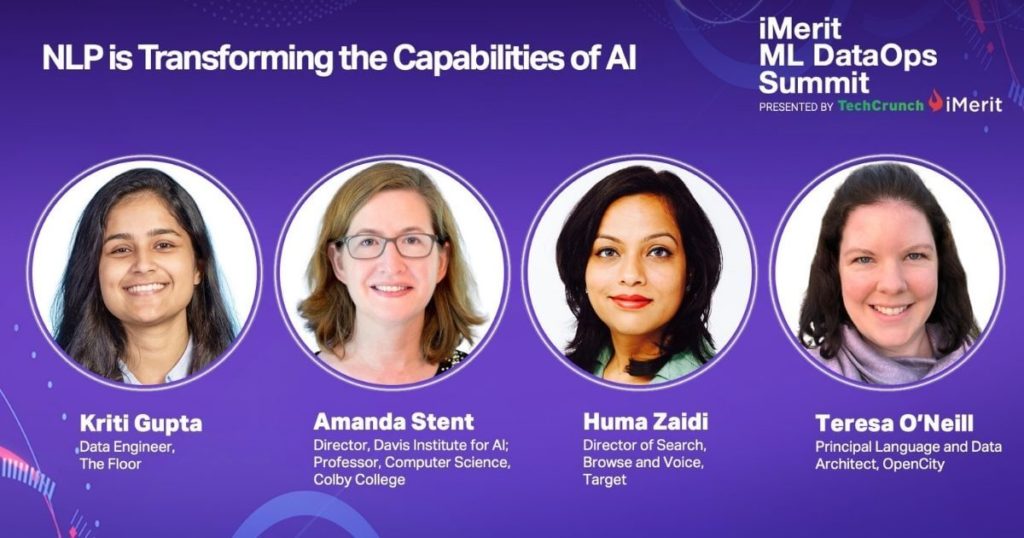
NLP’s Ever-Expanding Scope
Natural language processing applications have extensive use cases across almost all industries and verticals. As each industry has specific technical language, each NLP application must have a very well-defined scope that is able to understand terms and contexts specific to the use case.
NLP’s scope is also constantly expanding and evolving as the continuous evolution of languages and linguistics results in new and unique challenges such as new laws or procedures in healthcare, for example.
“Thanks to NLP, I can ask about the weather and even make calls while driving without really touching my phone. These use cases are expanding, and we need to understand how technology can support them.”
– Huma Zaidi, Director of Search, Browse and Voice at Target
Despite over 25 years since the release of Google search, over 15% of daily Google searches have never been seen before. Because of this, any NLP application that fully understands every Google search query ever would become obsolete in a short time. We see a difference in the search modality as well as 50% of overall Google queries are now voice-based. There is an inherent difference between how people phrase voice commands compared to typed commands.
Interfacing with Labelers
When defining a new product, we must think about how to include data labelers in the wider development team. In the initial stages of an AI project, the product team must define the problem space and the scope of the project. Afterward, the team must determine how the AI product will look when delivered – lower-level questions such as whether the product will include multimodality such as speech-to-text and computer vision.
Once the product is defined at a high level, the project needs to collect relevant real-world data. For example, an NLP project in the healthcare should collect data such as doctor’s notes, medical reports or diagnoses. The legal industry can include terms and conditions, contracts and agreements, and conversation transcripts and email exchanges. Once enough data is collected, these must be sent over to the specialized data labeling team to start the annotation process.
“Once the labelers see some kind of irregularity or trend they need to be able to communicate and define the definitions with the product team.”
– Kriti Gupta, Data Engineer at The Floor
During the annotation process, labelers have first-hand exposure to real-world data. They are able to identify trends, challenges and edge cases in the very early stages of the NLP development process. As such, data labelers must be included in the development ecosystem to forward their insights and observations to the development team. Regularly interfacing with labelers and truly integrating them in the wider ecosystem can help identify and overcome challenges and edge cases from the initial stages of product development.
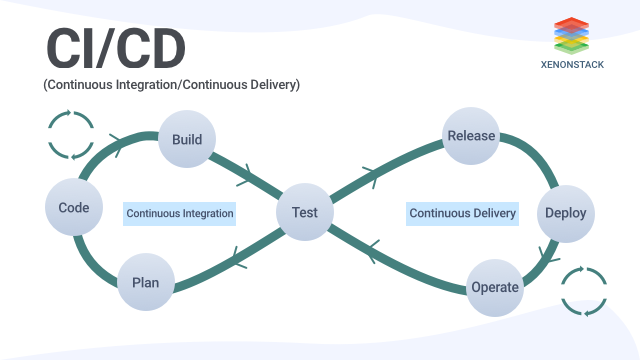
To facilitate that, a project management layer between the data labelers and the rest of the development team can streamline communications. A project manager can aggregate the input of multiple labelers to clearly communicate insights through a single channel. Similarly, a project manager is able to cascade communications consistently to the data labeling team to ensure that all labelers are fully aligned on definitions and instructions. Experienced project managers can translate any changes in scope into a wider project impact in terms of timelines and budgets.
“We are all learning and we’re going to be learning for a very long time, and the machine is going to be learning for a very long time.”
– Huma Zaidi, Director of Search, Browse and Voice at Target
Human agents can be included in-the-loop to ensure that NLP applications have high performance rates for commercial applications and to continue training the model on unseen data. Acting as the equivalent of a safety driver, a human agent can pick up the data where the NLP application has low confidence or fails, and then feed back the newly annotated data back into the NLP application.
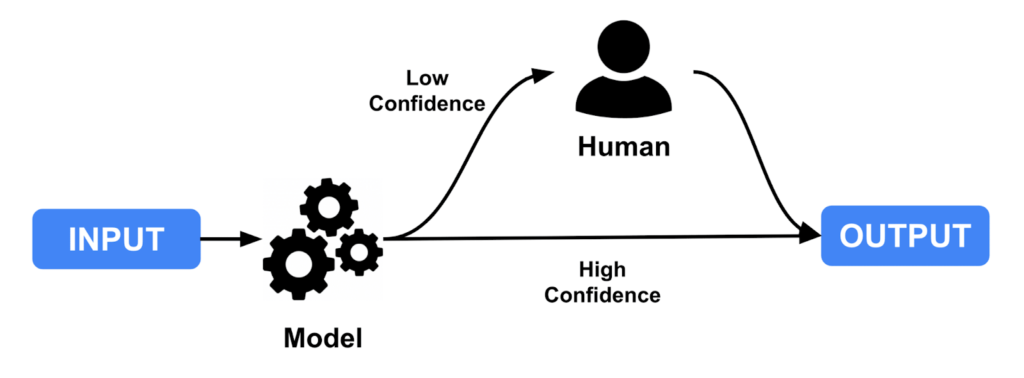
In Conclusion
Natural language processing is a non-exhaustible field as it is constantly adapting to the evolving linguistic landscape. We require a continuous stream of accurately labeled data and the ability to define and redefine the context in which language is used. Partnering with a data labeling service provider such as iMerit can ensure a consistent data flow to ensure that your NLP application is always up to date with new words, phrases and trends.