Developers in the early phases of their AI application lifecycle often use a “Goldilocks approach”, also known as the “just right” approach, to their first-generation AI applications. They want to apply AI in situations large enough to have noticeable benefit to their machine learning (ML), while avoiding larger applications with significantly more complex edge cases.
As the developer gains more experience and their ML models improve, they become eager to apply AI to large applications. Since the large applications need to handle more specialized edge cases, this drives demand for larger data sets and more data annotation.
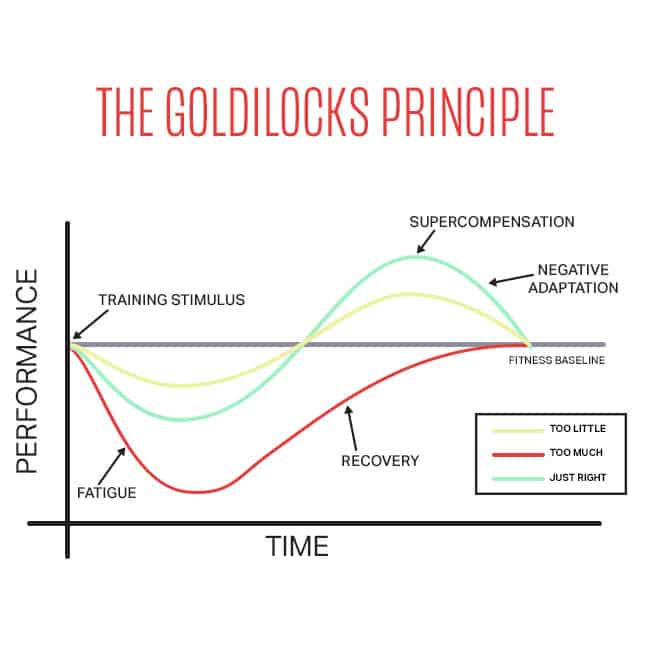
As we discussed in previous blogs, data annotation is often a necessary step to properly train your ML and AI.
AI Growth Can Be Seen Across Financial Services processes
The financial services industry understands AI’s potential for lowering costs, improving operational productivity and increasing financial performance. Even digital banking is in the midst of expanding AI capabilities to improve customer service with conversational AI.
“There will be winners and losers caused by the opportunities created from AI.”
Financial services are using Machine Learning/Deep Learning (ML/DL) to improve nearly every part of the balance sheet. For example, applying ML/DL to outward-facing customer sales and marketing activities can help drive new business with better personalization and customer satisfaction. Financial services organizations are beginning to see ML/DL applications directly improve core business areas from policy underwriting to claims management, pricing, investments and trading desk performance. Since it’s likely that ML/DL will usher in disruptive new business models, there is a big incentive for financial services organizations to participate.
“To the victor go the spoils!”. AI will provide a competitive advantage and financial service companies are in a race to apply AI to their businesses.
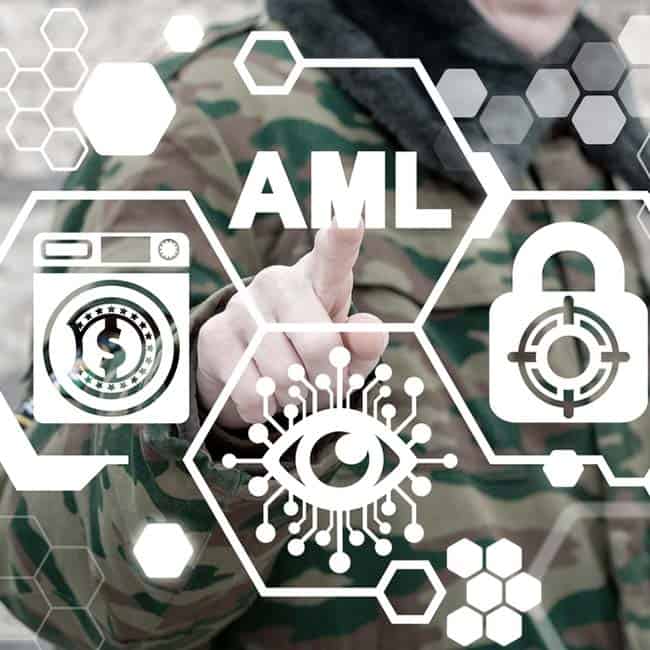
The rapid rise in the volume of digital transactions, growing amount of financial data, ever-increasing compliance regulations and rapidly increasing and sophisticated fraud techniques have left banks more vulnerable to fraud. The development of ML/DL technologies are arriving just in time. Consumer banking is applying ML/DL in exciting and cutting-edge ways to augment fraud detection and anti-money laundering. The level of manual work in traditional anti-fraud processes cannot cope with the new realities. Increased levels of automation are essential, and AI will be playing a major role in making that automation happen.
“AI is being used to detect fraud and money laundering with fewer false positives by doing a much better job of finding anomalies or behaviour patterns that indicate fraudulent activity.”
AI not only offers opportunities for new services, but also makes old processes more efficient and scalable. The use of human resources are expensive and each false-positive for fraudulent activity squanders the attention of scarce specialists where the resolution offers no real financial benefit. Gaining greater efficiency for how resources are applied within the organization is as important as the automation that is taking place.
Increasing Need for Data Annotation
Demand for data annotation services is being driven by the increasing number of AI applications, the increasing scale of applications and the proliferation of edge cases requiring specific training data.
In order to accelerate and scale AI development, AI developers should leverage data annotation service providers that have trained and retained a workforce of experienced annotators who can use the latest technologies. These teams have the ability to meet their customers annotation needs, which are increasing in both size and complexity. They are able to leverage their annotation capabilities in new ways and using capabilities first deployed in one industry to benefit other industries.
Leveraging Past AI Capabilities for New Situations
Example 1: Application of AI in “Factoring Loans”
An example of using annotation capabilities in new ways can be seen in innovative processes associated with “factoring loans”. A factoring loan is a financing solution that helps companies stabilize cashflow by unlocking the cash sitting in unpaid receivables and inventory. This process involves treating invoices and inventory as collateral for cash, allowing a company to receive cash in advance to fund operations. The physical inventory being shipped against those receivables becomes very important in securing the factoring loan and also an area of fraud. Monitoring and verifying inventory levels can be a challenge for the lender.
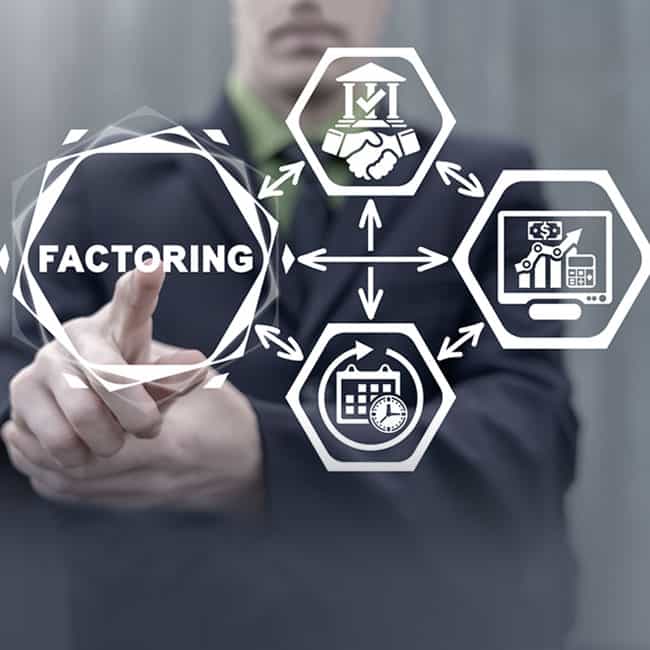
What if the inventory from which the loan is made against doesn’t exist? The ability for lender to annotate unstructured data from photos or videos helps them monitor inventory activity and manage risk. This allows the bank to issue more loans at better net profit by avoiding the fraud – and passing some of the savings to the receiver of the loan in the form of better terms.
Example 2: Applications of AI Retail Inventory
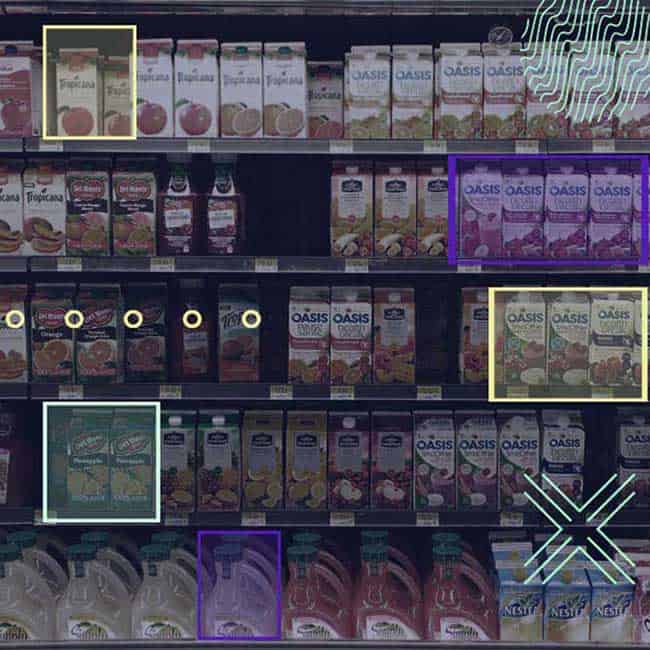
Here is another example of using annotation capabilities first deployed in one industry to benefit another. Photos or videos of items on retail shelves can be annotated to provide information on inventory counts on the shelves. What if a company wanted to take a commercial loan with inventory as collateral? Of course, the company’s Warehouse Management System provides the counts of each item in the warehouse’s inventory, but how do we know if those counts can be trusted and what’s on the shelf? AI and robotics can be utilized to annotate photos and video to determine how much inventory is on the retail shelves, giving them a stronger signal and confidence in what inventory is really out there.
Some Realities for Success with AI Stay the Same
AI applications typically combine the use of structured and unstructured data, with a sizable amount of data annotation, to bring everything together to create a new solution. This means that partnering with a very experienced annotation partner that is fluent across specialized annotation technologies and has the people and processes to create high-quality data, becomes a critical factor for success.