Since 1913, automobiles have been mass manufactured. The ensuing years saw the mass adoption of the automobile across the world. At first, people walked side-by-side on the roads with automobiles with few laws to protect them, nor the knowledge that such laws were necessary.
As fatalities mounted, so too did laws that were meant to prevent them. Police began handing out jaywalking tickets to prevent people from carelessly walking into traffic. By the 1920s road laws had become firmly established, and people found themselves walking on the sidewalk and using crosswalks in accordance with a stoplight system. It wasn’t long until people began fantasizing about cars that drive themselves.
Fast forward to today, and the world of automobiles and their corresponding laws are largely the same. But within R&D facilities and labs, an invisible revolution is taking place for automobiles. In an effort to continually improve the quality of life of citizens around the world, companies are working tirelessly to perfect an autonomous vehicle that is capable of obeying street laws, identifying obstacles, and removing human error from driving altogether.
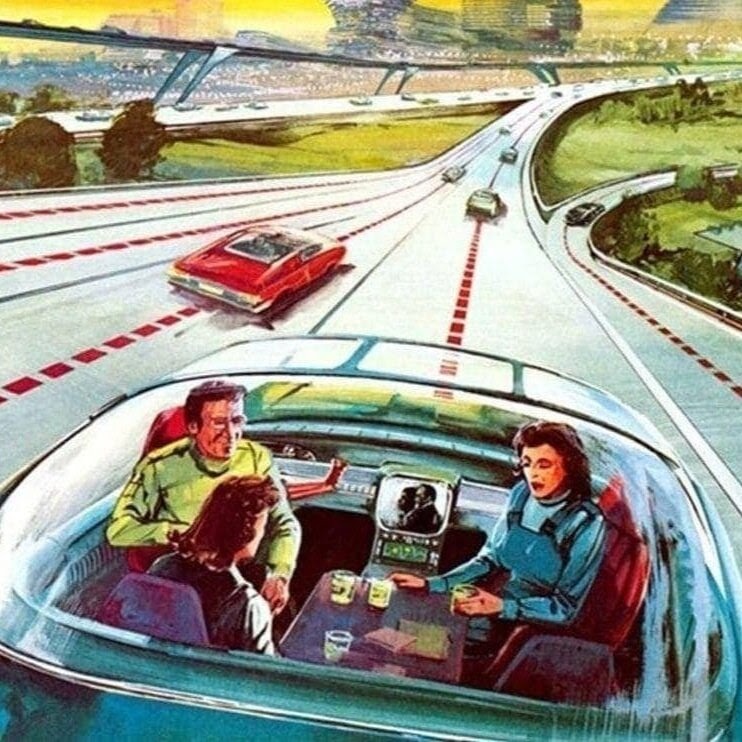
Edge Scenarios
Autonomous vehicles are a hot subject today. The biggest names in the automotive industry are regularly showcasing the rapid progress they’re making in developing an autonomous driving machine.
While the progress of AV technology has been substantial in the past decade, the technology still faces a massive hurdle that’s preventing mass adoption. This hurdle is known as an “edge scenario,” which is a unique situation or unusual occurrence in which an autonomous vehicle is unable to properly address or identify an unusual obstacle, circumstance, or happening on the road, which can result in an accident or fatality.
Varying Complexity
An infinite number of scenarios can take place on the road. While the human eye can account for random developments on the fly, autonomous vehicles must be explicitly trained to account for these same obscure developments. To address edge cases, autonomous vehicles require special design considerations in order to handle these developments securely.
As data is largely generated manually for the training of autonomous vehicles, it is challenging to train vehicles to perform for largely implausible happenings that still take place every day on the road. We have known for a long time that it is difficult to build a life-critical system that is capable of perceiving and adequately addressing roadside developments, laws, and subtle deviations from the norm these ML models are trained upon.
Environmental perception refers to developing a contextual understanding of the environment, such as where obstacles are located, detection of road signs/marking, and categorizing data by their semantic meaning. A trained model can predict many edge cases, and edge case training can make the autonomous system more robust under novel operational conditions.
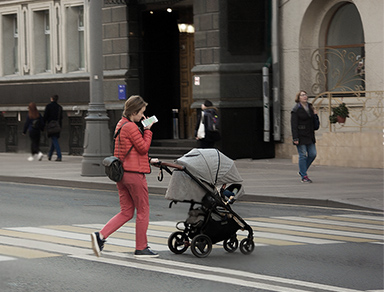
For instance, a road sign with an image of a bear can be confusing for an autonomous vehicle. An AV may treat it as an object and stop abruptly. A woman with a pram on the road might not be identified by the car. It is crucial to factor a balance between analysis and field experience because an edge case like a lion on the road could be a single point of failure if not trained.
Each country, city, and landscape presents its own unique set of edge cases. Anticipating and training for these challenges is an ongoing challenge all on its own. Context is an essential element when defining the nature of any roadside development or sign. For example, every country/city’s police department has its own distinct appearances, and every city/country has its own set of unique road laws. While lions on the road may be commonplace in certain parts of the world, they’ll almost certainly never take place within another.
The most significant safety benefit of an autonomous car is that it is not a human—it is designed to follow all traffic laws, not speed, and to be indestructible by minor things like text messages or flashing phone screens. AVs can also detect what humans can’t—especially edge cases—and respond more rapidly to prevent a collision, at least in theory. Accurate labeling of edge case data helps push this from theory to reality.
The iMerit Solution
iMerit takes great care to document each and every edge case encountered in order to continually anticipate them in future cases and help their clients overcome them. When working on large-scale data annotation projects, iMerit employs an expert team of data labelers who’ve worked on other autonomous vehicle projects in the past.
iMerit’s multi-tiered approach to data labeling forms a clear, structured workflow and communication to ensure optimal data labeling. The expertise these data labeling teams bring to the table includes decades of experience working with and solving edge cases, particularly through anticipating them based on past experience.
A feedback loop is employed upon completion of all data annotation, where the client can see if the annotated data will meet the needs of the final use case. During these meetings, iMerit’s data labeling teams can explain how their approach accounts for certain edge cases that this company may have already been experiencing. In the event that data labeling is deemed inadequate, the process starts all over to account for any discrepancies.
Once the annotation is up to par, the data will then be sent to the Quality Control (QC) team, who will work with iMerit’s data labeling experts to ensure tasks are being carried out accurately and on time. The QC team can then learn from the data labeling experts how their approach is addressing the edge case.
iMerit’s teams can also help companies understand where data is lacking that could help address a certain edge case and inform the subsequent generation of such data. This can drastically reduce costs, as data generation on its own can be as complicated, laborious, and expensive as the edge case itself.
Conclusion
Edge cases are a massive hindrance to the mass adoption, safety, and efficiency of autonomous vehicles. Anticipating and addressing them is an essential element of success for any company hoping to be successful in launching their autonomous vehicle. With the right workflow, talent, and understanding of a region’s given edge cases, overcoming edge cases can be done effectively.