It’s perfectly normal to reassess if your data-labeling methods are meeting the needs of your organization. For anyone who has labeled data using in-house teams, you’ve likely experienced a range of challenges including cost, time, and even manpower deficiency. In this piece, we focus on the telltale signs that it may be time to outsource your data labeling to a professional team, and why it will save you a big headache in the long run.
Four Signs it’s Time to Hire Professional Labelers
You can determine whether you will benefit from outsourcing your data labeling activities if you identify some of the following situations:
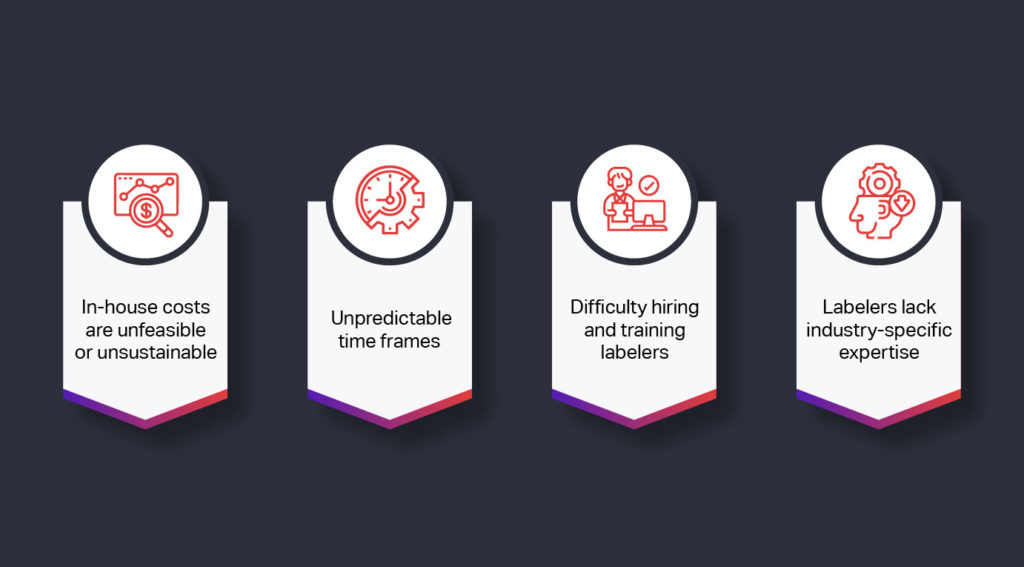
1. In-house costs are unfeasible or unsustainable
Labeling data in-house is especially expensive in advanced economies, where worker wages are high. In our article 5 Steps to Guarantee a Successful Data Labeling Program, we’ve offered the following example:
1000 images * 2 hours spent per image * $9.35 local minimum wage = $18,700.
For larger and larger datasets, these costs can scale to the point where it is no longer feasible to continue labeling in-house. This is the most common pain point that in-house data labeling produces.
2. Unpredictable time frames
When working with an internal team, overall performance can suffer as labelers leave their role, new hires are brought onboard who require training, or resources are reallocated to different projects. Outsourcing to a reputable third-party vendor guarantees delivery dates through contractual agreements, which stipulate that data will be delivered at specific time intervals with an acceptable quality level.
3. Difficulty hiring and training labelers
If your in-house labeling team has shrunk or is not large enough, recruiting new labelers isn’t always feasible. This is because new hires require training to produce acceptable quality labels.
4. Labelers lack industry-specific expertise
Some industries – such as healthcare and medicine – require a level of subject-matter expertise from the labelers performing the annotation. These labelers should also have an intimate level of technical understanding about the datasets they are working with. In instances where the in-house labelers do not have these skills and recruitment opportunities are limited, the project may be better served by working with a labeling company whose data annotators have industry-specific skills.
At what stage of the project should you outsource data labeling?
Assuming you’re experiencing any of the above warning signs, hiring professionals to label your data sooner rather than later is likely your best bet. But how feasible is it to outsource data based on the phase of your project?
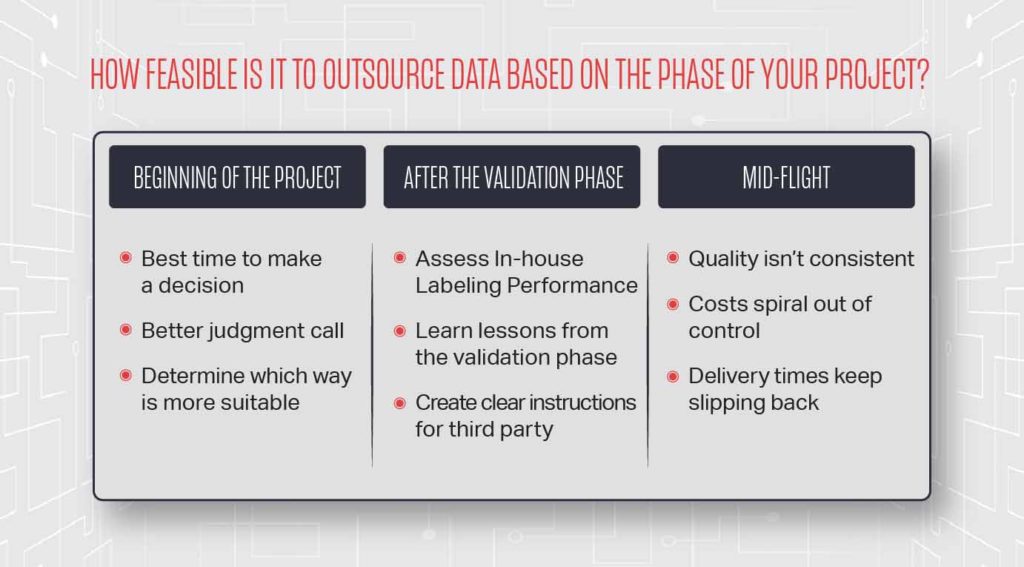
1. Beginning of the project
This is the best time to make a decision. If in the past you’ve experienced pains in an AI project around data labeling, then this is the time to make a judgment call on whether to hire professional data labelers or not. Some questions that you can ask to determine which way is more suitable for you can be the following:
- Do you already have an existing in-house labeling team?
- Does your labeling team need industry-specific training?
- Do you have any budget constraints for the labeling activities?
2. After the validation phase
Whether planned or unintentional, this is an excellent point to determine whether you can continue labeling in-house or partnering with a labeling solution provider. Considering that only about 20% of the dataset is used for validation, this proof-of-concept labeling exercise can definitely be done in-house without mounting concerns around costs or turnaround times.
Upon completion of the validation phase, engaging with a data-labeling service provider can considerably accelerate the process and simultaneously reduce costs. Using lessons learnt from the validation phase, you can create a set of clear instructions for the third party and thereby mitigate their learning curve.
3. Mid-flight
Keeping in mind the sunk cost fallacy, if you notice during your project that costs are spiralling out of control, your in-house labeling team is burnt out, quality isn’t consistent, or delivery times keep slipping back, we recommend reaching out to a third party labeling provider. It can be very tempting to continue working in-house just because of all the effort already invested despite any negative consequences.
Types of Data Labeling
There are multiple ways to outsource your data labeling, with the best avenue depending largely on your needs and requirements.
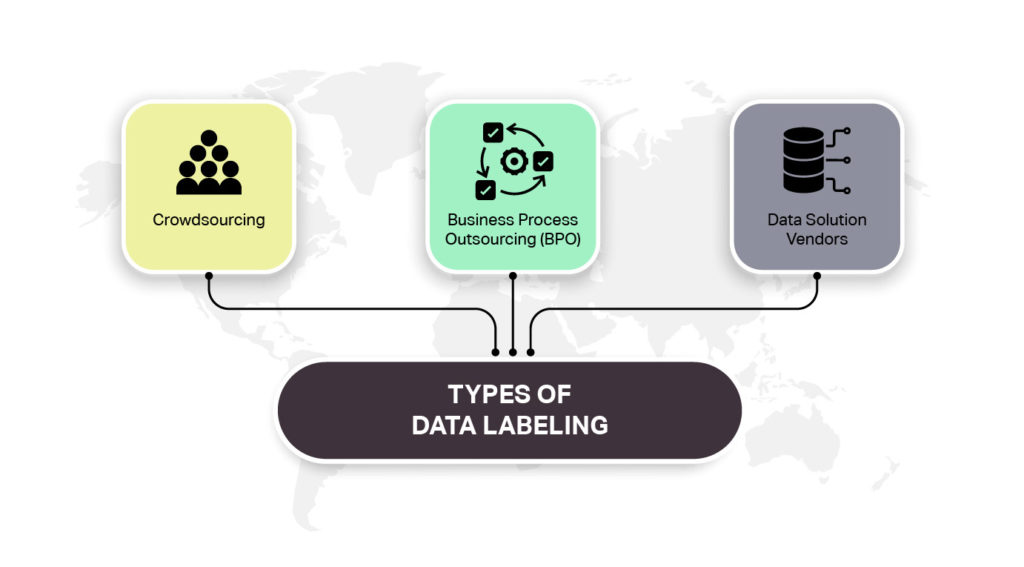
1. Crowdsourcing
Perhaps the cheapest and fastest way of gathering data, crowdsourcing is an outsourcing method in which small tasks are delegated to large numbers of people to perform data labeling tasks. Crowdsourcing projects are typically mediated through third party platforms such as Amazon Mechanical Turk. The process is similar to a recruitment platform, where a client – in this case an AI/ML company – posts a task on a platform which is then carried out by independent workers – in this case data labelers.
Considering the low cost and ability to scale, crowdsourcing is an excellent platform for simple tasks like generic product categorization or age categorization. However, multiple studies [1] [2] have consistently shown that crowdsourcing falls short with more complex tasks. Compared to a managed workforce, crowdsource ranks lower in accuracy on all evaluated tasks such as sentiment analysis, transcription, extracting information, and classification.
2. Business Process Outsourcing (BPO)
In this context, we refer to Business Process Outsourcing as delegating the process of data labeling to a non-specialized third party. A wide variety of BPO vendors offer a range of services such as data entry, customer service support, payroll, and even data labeling. These vendors have a skilled workforce that can deliver high quality business process services.
Compared to crowdsourcing, BPO vendors deliver data labeling services with higher accuracy for a variety of reasons including basic training for the workers, the opportunity for workers to specialize in one area, and a formal working environment.
3. Data Solution Vendors
These types of vendors focus exclusively on providing high quality data labeling services. By leveraging their extensive data labeling experience, these specialized vendors can provide you with high quality annotations while also supporting your wider AI/ML project needs. These vendors can help you estimate how much data you need, establish delivery volumes and time frames, as well as work with your development team to define good practices and guarantee quality assurance.
Having worked on a multitude of AI projects, these vendors have the required expertise to identify common issues and devise a strategy to ensure a smooth project.
Final Thoughts
As previously stated, it’s perfectly normal to constantly reassess how your data is being annotated. In many cases companies learn to live with their pain in hopes to eventually overcome it organically. While feasible, this comes at a high cost that can be devastating.
If funding or manpower are your primary challenges and your project is simple, then crowdsourcing will likely be your best option. But if your industry demands trained labelers to accurately annotate your data, then look no further than a data service provider like iMerit today. By leveraging advanced tools, machine learning algorithms and workflow best practices to enrich, annotate, and label large volumes of unstructured data, iMerit can help take your AI or ML project to the next level.