From diagnostic assistance to patient engagement, AI in healthcare is proving beneficial for patients and healthcare professionals alike by increasing efficiency and reducing significant cost. Expert annotation is the key to creating high quality training data to power AI-enabled healthcare. Here are some of the top AI applications in healthcare and the data annotation that plays an important role in their development.
Virtual Assistance
Virtual Assistants
Remote monitoring sensors are being leveraged to power 24×7 virtual nursing assistants which can accelerate the identification of illness, monitor health status, schedule doctor appointments and provide medication reminders, bringing value to both patients and doctors.
Data Annotation: Key Point Annotation, Facial recognition, Gesture recognition for Sensors; Data extraction from patient wearables
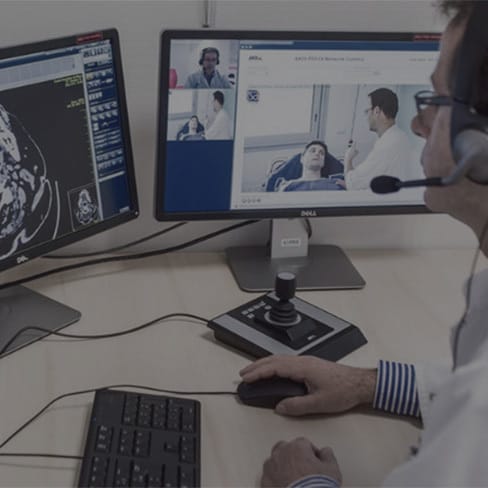
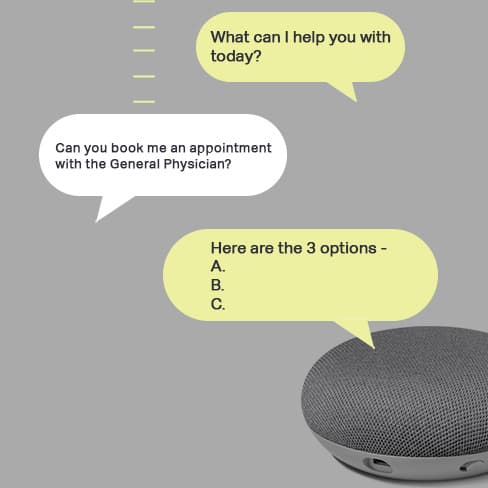
Conversational Bots
Conversational AI solutions have the potential to make life easier for patients, doctors, nurses and other hospital and clinic staff in a number of ways. Conversational AI use cases in healthcare include symptom checking, escalation of emergency cases, scheduling doctor appointment and patient engagement. A recent pilot study is exploring how conversational robots can be used to support individuals with Alzheimer’s Disease.
Data Annotation: Sentiment/Intent Analysis, Entity Recognition
Diagnosis
Medical Image Annotation for diagnostic support
Human error is a problem in CT scan analysis. Artificial intelligence will bring to market many new categories of CT scan, MRI and X-Ray analyses and show major improvements in speed, accuracy and costs associated with the analyses. For instance, AI can detect pneumonia caused by COVID-19 in chest CT scans or in the case of IVT treatment, AI can perform embryo classification with an accuracy higher and faster than embryologists can perform..
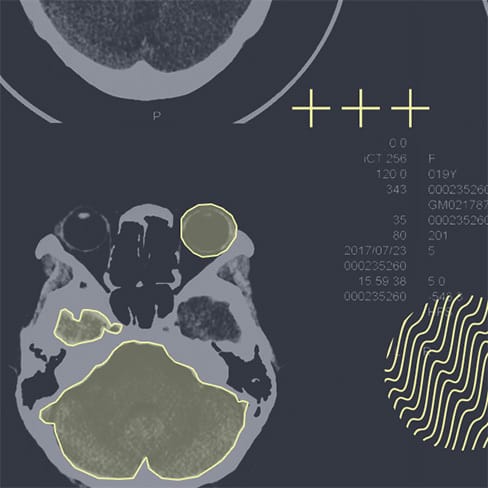
Data Annotation: Annotation of Medical images such asMRI, and X-ray, and CT scans for pneumonia diagnosis and accurate embryo classification for IVT treatment
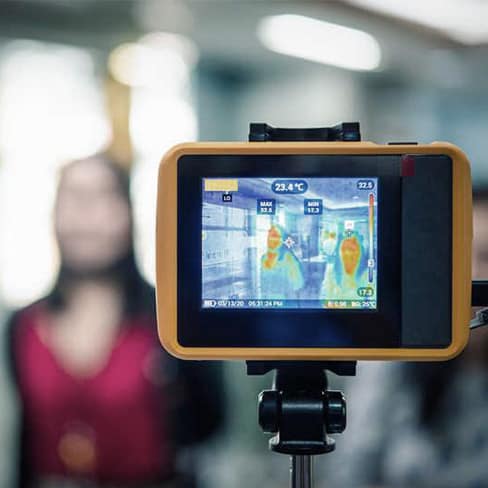
Thermal Image annotation for early diagnosis
Artificial Intelligence over thermal images are being used for early detection of breast cancer as thermal images can visually display the amount of infrared energy emitted by a tumor. AI can also quickly parse through many people at once to identify people with high temperatures. This can help to identify symptomatic individuals and alert the person to seek additional screening.
Data Annotation: Thermal image annotation for early diagnosis of breast cancer and quick identification of pedestrians with high temperatures.
Medicine
Pattern Recognition for drug development
Through machine learning algorithms, drug development can be improved by advancing the search for chemical and biological interactions. This will help bring new pharmaceuticals to market quicker. Huge volumes of data from sources such as research papers, patents, clinical trials and patient records are taken into account for drug discovery. It generates billions of known and inferred relationships between biological entities such as genes, symptoms, diseases, proteins, tissues, species and candidate drugs.
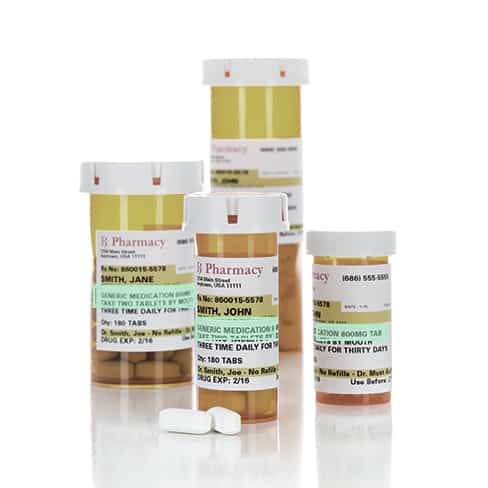
Data Annotation: Natural Language Processing to recognize entities, attributes, and understand relationships between factors
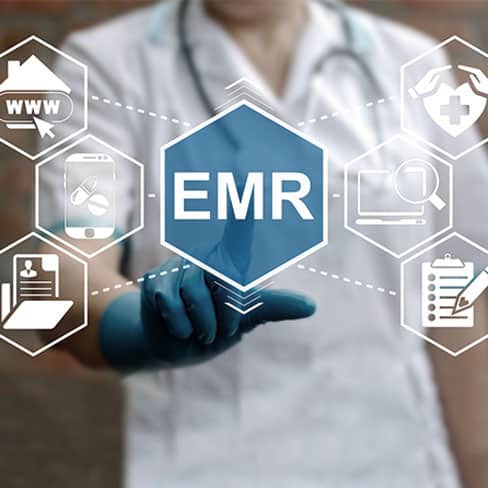
Data Extraction for personalized drug treatment
Clinicians use a personalized medicine approach to find an appropriate drug combination along with a dosing strategy over time based on the individual’s data. This is more relevant in the treatment of diseases like cancer, where multiple drugs are administered simultaneously to the patients. Studies found this approach to be superior when compared to the drug and dosage decisions based on clinical trials representing a sample population.
Data Annotation: Digital Radiology, Labeling bio-images (MRT and CT scans), electronic medical records (EMRs), health claims data from insurance companies, and data from wearable sensors and mobile health applications.
Surgery
Precision annotation and labeling for robotic surgery
Just as lane assist and collision detection ushered in the first generation of autonomous features in vehicles, computer vision driven autonomous features in robotic surgery are poised to make surgeons better and surgery safer. To accomplish this experts are working with specialized annotations teams to label critical structures in millions of frames of surgical videos.
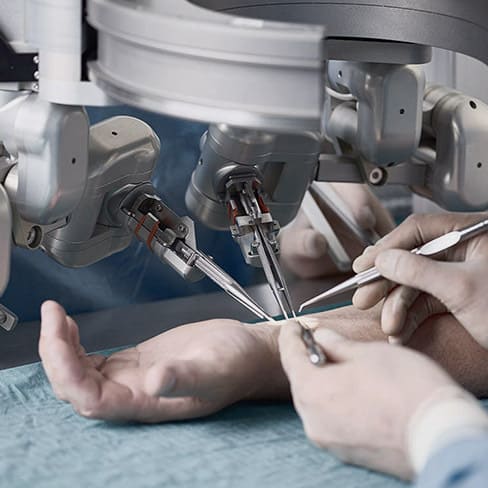
For example, one such project required pixel level annotation of the various anatomic structures within a Robotic Coronary Artery Bypass Graft or CABG video. To accomplish this, the team utilized a combination of modularized elearning lessons with classroom sessions to augment core skills in 3D spatial reasoning and precision annotation with the necessary clinical knowledge.
Data Annotation: Lesion detection, Phase identification
Patient Follow up
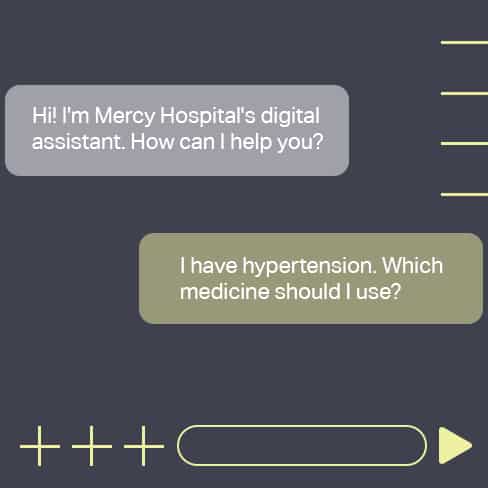
Entity Recognition to power Chatbots
The three main areas where chatbots can be particularly useful include diagnostics, patient engagement outside medical facilities, and mental health. Healthcare payers, providers, including medical assistants, are beginning to leverage AI-enabled chatbots to simplify patient care and cut unnecessary costs. Recently, Northwell Health, an AI company developing chatbots that will help patients navigate cancer care, says more than 96 percent of patients who used its post-discharge care chatbots found it very helpful, demonstrating the increased client engagement.
Data Annotation: Dissecting text and audio in medical records, digital documents, and clinical trial data, Intent and conversation analysis
Transcription for record management
To ensure that patient care is adequate and nothing is missed, accurate patient medical records are an important part. AI-powered medical scribes are able to bring the quality and experience of a human medical scribe, but at the cost and scalability of a dictation service.
Data Annotation: Audio and Text Transcription to digitize important speech or handwritten information from patient sheets or medical records.
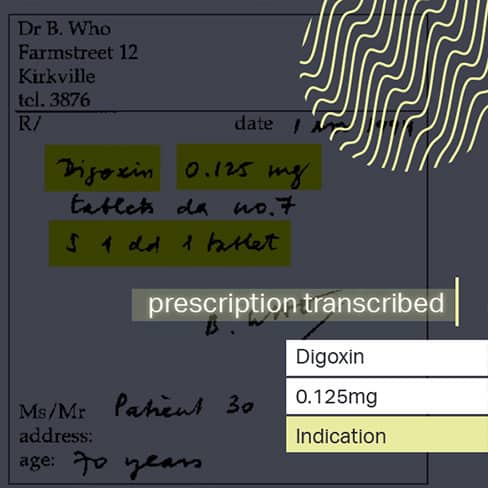